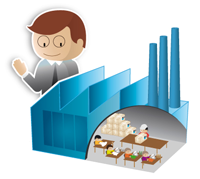
In China, in many product lines, a factory that makes 95% good products is considered a good factory. And its managers will not feel ashamed.
Now, let’s look at it another way. A factory that ships 5 million pieces a month, at a 95% quality rate, ships nearly 3 million bad pieces a year to its customers. Not quite the same story. There is no reason to feel satisfied. They should work hard at raising the bar.
And, from a customer’s point of view, it is not pretty either. Let’s say you purchase 20% of that factory’s output. You will receive more than half a million bad pieces a year. You should keep a close eye on their production.
Statistical tools can help change people’s perspectives in many ways. I listed a few examples below.
Having the production and the quality departments work together
Production and QC people tend to fight instead of working together. For example, an inspector finds a problem and asks to stop a process. But the production people (who have targets about their workshop’s output) will say “show me a defect now” and “we just checked it together and we couldn’t see any problem”.
In certain factories, statistical process control can be an excellent solution. It gives simple rules for stopping a process and having a close look at it. And ideally it involves production operators rather than quality control technicians.
Expecting some level of variation
Nearly all Chinese factories have very poor process control. It means luck plays a large role in the result of their production efforts. (Put nicely, “random elements” affect the quality level, the amount of scrap, as well as timelines in a significant manner).
If a quality auditor estimates that the quality level is 95%, and if a few shipments in a row are at a 99% quality level, one bad shipment will come up soon. You can count on it. The concept behind this is called “regression to the mean” and can be considered a law of statistics.
A statistician joked that, if the topic of regression comes up in a court of justice, the side that must explain regression to the jury will lose the case. So I won’t try to explain it in depth, but Wikipedia can help.
Distrusting cause-and-effect explanations
Let’s say a particularly bad shipment triggers long meetings with the factory’s management and hefty penalties. And this shipment is followed by a markedly better production run. It means the buyer’s response was appropriate and the supplier paid much more attention to production quality, right? I would not bet on it.
Again, regression to the mean is at work here. A particularly bad shipment (way below average) is usually followed by a shipment that is better (and closer to the average.)
No such thing as an auditor’s “golden fingers”
QC inspectors usually work on the basis of statistical tables. But most auditors don’t. For example a quality auditor will pick three samples of warehouse incoming records and will then ask for the corresponding incoming QC records. If the factory is unable to provide two of the three requested documents, they will probably say “you took just the wrong ones, but if you check more you will see it is a very isolated problem”.
What is the chance that the auditor picked those two documents out of hundreds of files, and that those two are the only ones missing? People well versed in statistics will say it is close to impossible.
Now, if you have read this article until the end, try to explain this to your Chinese suppliers… The challenge is often to translate these concepts in a very down-to-earth manner.
Do you agree?
No comments:
Post a Comment